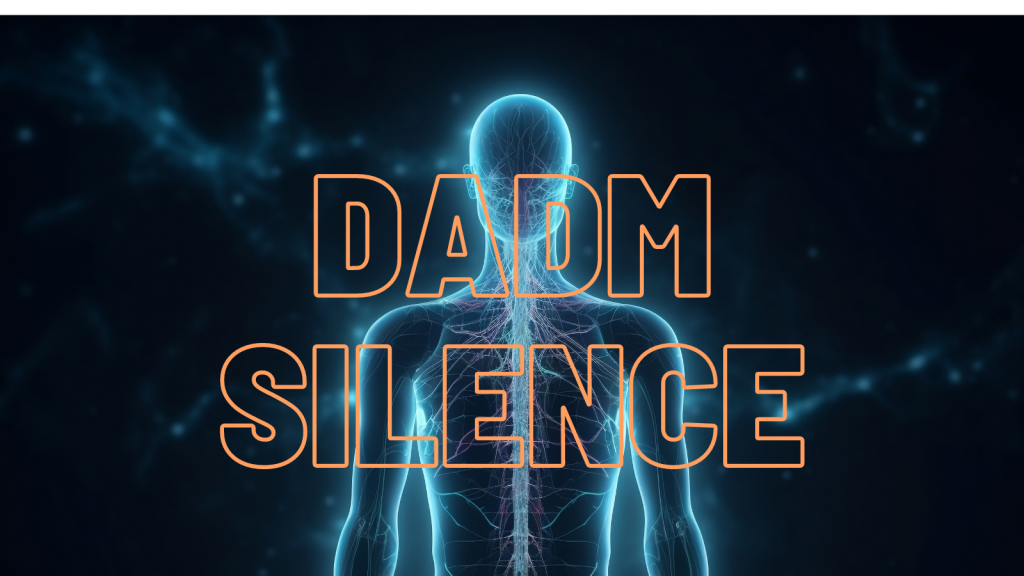
The DADM’s Noticeable Silence: Clarifying the Human Role in the Canadian Government’s Hybrid Decision-Making Systems [Law 432.D – Op-Ed 2]
This is part 2 of a two-part series sharing Op-Eds I wrote for my Law 432.D course titled “Accountable Computer Systems.” This blog will likely